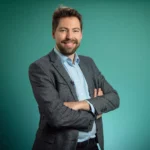
iMaster NCE: The Art of Optical Automation
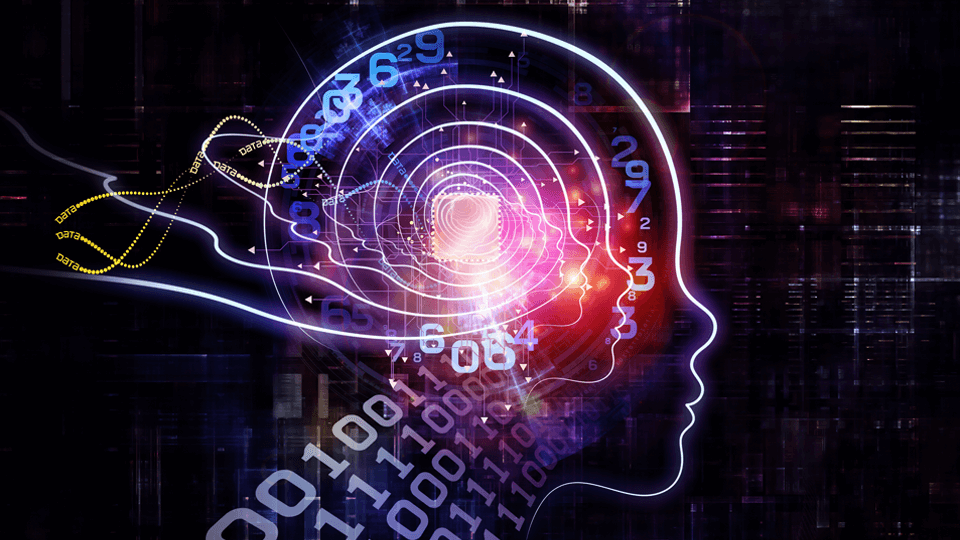
ByDr. Christopher Janz
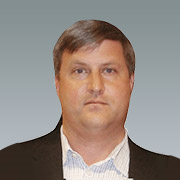
Obstacles to Optical Network Automation
In talking about obstacles and impediments to optical network automation, I will focus very closely on issues specific to the optical network. To organize my points I will use the well-known, four-part functional architecture for automation loops: data, analysis, decision, and action. Data relevant to analysis is collected, then processed using analytics algorithms to produce the actionable intelligence on which optimized operational decisions can be made. The outputs of such decision processes yield both automatable and optimized operations outcomes and processes.
First: Historically, optical networks has been very weakly instrumented. Reported stats have been dominated by pre- and post-FEC BERs, which do not provide a good view of optical impairments or breakdown of optical performance metrics. Things like linear and nonlinear noise and filter impairments have not been visible.
Second: Historically, optical performance modeling, such as for connection performance prediction, has not been attempted in real time on operational systems. Physical modeling is applied in offline planning environments, but is cumbersome, time-consuming and approximate. It does not benefit from network-specific data, for example, fiber parameters and models specific to deployed or to-be-deployed devices. No real-time performance prediction capability supports real-time operational decision-making.

Third: Optical network operations depend on decisions such as new service and protection path selection and allocation, batching and sequencing of provisioning operational steps, and fault localization and classification. Without accurate performance assessment on operating optical services, and performance prediction on as-yet-unprovisioned optical services and configurations, these decisions are made largely blind. Additionally, configuration planning for new services and protection and restoration facilities is cumbersome and not geared towards resource optimization.
Consequently, very little automation of operations has been possible on optical networks and substantial operational risks and inefficiencies have accrued on many processes. Provisioning and restoration operations are risky and can be slow; new service and dynamic restoration configuration planning are heavily manual and risk-laden; fault management is manual, slow and reactive. All of these things keep the optical network quasi-static and well-separated from service delivery value. Lack of performance knowledge requires heavy excess margin provisioning, leading to significant underuse of inherent network capacity.

Addressing Obstacles to Automating Optical Network Operations
How are the historical obstacles to optical network automation being lifted?
First: a new generation of directly optically relevant instrumentation is proliferating in networks. Coherent receivers are signal processing platforms that already determine and annul certain polarization-related impairments. Recent research has developed algorithms that allow for linear and non-linear noise to be directly measured, separated and reported. Furthermore, cost-effective, embedded OTDR permits continuous measurement of fiber parameters, including distributed and point losses. Embedded power spectral measurements permit direct reporting of the evolution of channel powers and evolving filter shaping throughout the network. We’ve also developed methods for detecting co-cabling of fibers to help eliminate inadvertent shared link risk groupings, as well as methods to reliably identify fiber types.
Analytics modules attached to off-board domain controllers (in our case, iMaster NCE) receive data from this instrumentation and host complexes of new device and system models. These models, for EDFAs, are developed using supervised machine learning and are highly accurate: specific to amplifier type and even instance, with the support of some point-of-manufacture measured data. Further models have been trained, for example, to translate filter shapes to margin penalties. Measured data and models work together flexibly to yield key actionable intelligence: margin on operating and prospective connection services.

Supported and informed by this information, key decision processes are being implemented in software. New, fast-executing heuristic algorithms can find globally resource-optimized provisioning configuration solutions, applied, for example, to batches of new service demands or pre-planned dynamic restoration responses, leveraging all available degrees of freedom – route, spectrum, modulation, coding and baud rate – and respecting restraints such as latency caps. Performance prediction assures the operational integrity of solutions. Time-based analysis of telemetry permits prediction of worrisome degradation and soft or hard faults.

All of these capabilities are in hardware and software, such that information-based optimized operational decisions are generated continuously and susceptible to fully automated instantiation in different operational applications. We will consider some of those in the next section.
Automating Operations
Now let’s look at a few applications and use cases that are improved and rendered susceptible to automation in optical networks through advancements in data, analysis, and decision hardware- and software-based processes.
Optical margin compression: With accurate knowledge of margin – in both measurement and prediction senses – we can begin to liberate the stranded capacity on optical networks that comes from excessive margin reservations. We can first move from static operation with end-of-life margins, to interval-based planning with reduced margins, and then eventually to dynamic quasi “margin-less” operations. With accurate knowledge of margin and heuristics to quickly and efficiently find globally resource-optimized allocation solutions – with tunability among potentially all of route, spectrum, modulation, coding and baud rate – we can consistently increase effective network capacity when compared with static and fixed parameter transponders. In the end, a dB of margin gained through accurate margin assessment is as valuable as a dB gained through improved modulation and coding.
Provisioning automation: With data-supported real-time modeling capable of accurately predicting power, noise and margin evolutions through configuration changes – either channel add/drop/re-route or protection/restoration – it becomes possible to find batching and sequencing combinations in any given case that maintain channel performance integrity throughout a provisioning operation. These execution recipes can be directly put into action for full automation.

Fault preemption: The tracking and extrapolation of optical performance evolution supports prediction of soft and hard impairments and faults, supporting preemptive action e.g., through protection switching and then proactive (vs. reactive) planning of repair operations. Action tickets can be generated automatically.
Risk mapping: The accurate assessment and prediction of optical performance can help identify performance risks on existing optical services, on planned new or reconfiguration optical services, and on planned restoration configurations and services. Degradation, failure, and other scenarios can be hypothesized to assess risk scenarios.

Dynamic restoration optimization: The identification of performance risks on planned restoration configurations triggers determination of a new, resource-optimized restoration map, using fast-executing optimization heuristics, supported by performance prediction to assure the integrity of proposed post-restoration configurations. These revised, optimized restoration maps can be directly pushed into active status without human intervention.

All of these use cases, and many more supported by the foundational capability described, make the operationally automated optical network a flexible and dynamic platform that can more effectively participate in the dynamic delivery of services.
Huawei Optical Automation Art: iMaster NCE
The heart of Huawei’s optical automation solutions is our domain control and management platform, iMaster NCE. This platform supports standard open APIs northbound (particularly, the IETF ACTN models) for control abstraction and configuration, as well as performance data exchange. It incorporates a data platform as a repository for network instrumentation data. It includes various analytics modules, a key such module being OPE – Optical Performance Engine. OPE works with instrumentation data, network and service topology, and inventory information, and includes models and algorithms to provide optical performance – margin – on request and with respect to both operating and hypothetical service configurations and network states. It can deliver such answers at high volume, supporting demanding concurrent applications.

Rather than relying on “black box” machine learning study of whole networks, OPE uses highly-accurate atomic models for components and resulting impairments to determine margins. It can generate accurate results at any stage of a network life cycle. It provides a basis for real-time configuration control optimization, planning tools, and fault prediction.
Illustrated are user-facing screens showing assessed margin histories on operating services, along with risk mapping “flags” highlighting assessed performance risks on particular existing services in steady state on prospective reconfiguration of services.
OPE is a foundational capability that will permit iMaster NCE to support many more applications driving operations automation and operational outcome improvements.
Read more about the use cases and success stories of the Huawei iMaster NCE.
Co-Authored by
Peng Cai, Senior Marketing Expert, ICT Products & Solutions
